The poor performance of the best models in computer vision - when tested over artworks - coupled with the lack of extensively annotated datasets for cultural heritage (CH), and the fact that artwork images depict objects and actions not captured by photographs, indicate that a CH-specific dataset would be highly valuable for this community.
DEArt was created with these challenges in mind. DEArt is a dataset for object detection and pose classification meant to be a reference for paintings between the 12th and 18th centuries. It contains more than 15,000 images, about 80% non-iconic, aligned with manual annotations for the bounding boxes identifying all instances of 69 classes as well as 12 possible poses for boxes identifying human-like objects. Of these, more than 50 classes are CH-specific and thus do not appear in other datasets; these reflect imaginary beings, symbolic entities and other categories related to art. Additionally, existing datasets do not include pose annotations. Our results show that object detectors for the CH domain can achieve a level of precision comparable to state-of-art models for generic images via transfer learning.
We are extending the annotations of DEArt with descriptions of the visual content for the images. To train a good description generation model, we are requiring each image to have 4-5 descriptions. This annotation set is being updated through a still-ongoing crowdsourcing campaign on the Zooniverse platform. Through this campaign, more than 6,000 images have been fully annotated with 4 or 5 descriptions. SGoaB will continue running this campaign at least until the 7,543 images uploaded on the platform are fully annotated.
Our paper, "DEArt: Dataset of European Art", has won the Best Paper Award at the Vision for Art (VISART VI) Workshop at the European Conference of Computer Vision (ECCV).
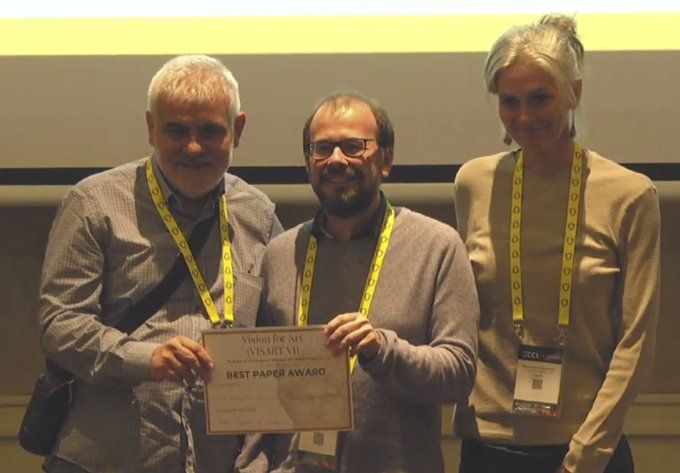